SSD-HIP: Semi-Synthetic Dataset for Hamstring Injury Prediction Using Electrical Impedance
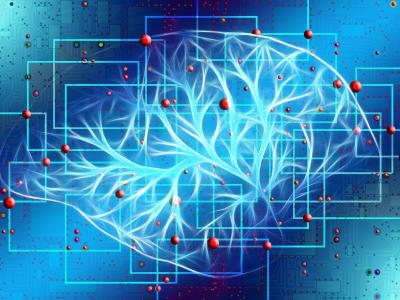
- Citation Author(s):
- Submitted by:
- Reem Shehayib
- Last updated:
- DOI:
- 10.21227/b57z-my16
- Data Format:
- Links:
- Categories:
- Keywords:
Abstract
Hamstring Injuries (HSIs) are common among athletes and necessitate extended rehabilitation before Return to Sport (RTS). Post-injury, athletes undergo physical examinations, which often fall short in assessing injury severity or guiding rehabilitation. Therefore, imaging techniques such as Magnetic Resonance Imaging (MRI) are used to evaluate the injury more comprehensively, aiding in the assessment of optimal rehabilitation and RTS timelines. Given the significant impact of HSIs on athletic careers, early prediction is essential. This dataset uses Electrical Impedance Tomography (EIT) for HSI prediction using the EIDORS software (Electrical Impedance Tomography and Diffuse Optical Tomography Reconstruction Software).
This semi-synthetic dataset was created using MRI scans of patients with hamstring injuries. The dataset was developed by mapping the boundaries of the hamstring muscles (semimembranosus, semitendinosus, and biceps femoris) with EIDORS. EIDORS generated EIT voltage measurements by defining muscle boundaries and setting appropriate properties, forming the basis for the dataset. The development of this dataset was crucial, as no existing datasets covered EIT measurements for various hamstring muscle conditions. ML models can be employed to validate the semi-synthetic dataset by distinguishing between injured and healthy hamstrings.
Instructions:
There are 16 features: rms_feat, integral_signal, zero_crossings, waveform_length, auto_regressive_coefficients, temporal_moment, avg_amp_change, min_value, max_value, variance, log_detector, linear_env, v_order, modified_mav, skewness, fourier_series. These features quantify the signal generated using EIDORS.
The label is under the title 'status', where '0' signifies healthy and '1' means injured.