Real Time Traffic Congestion Management System
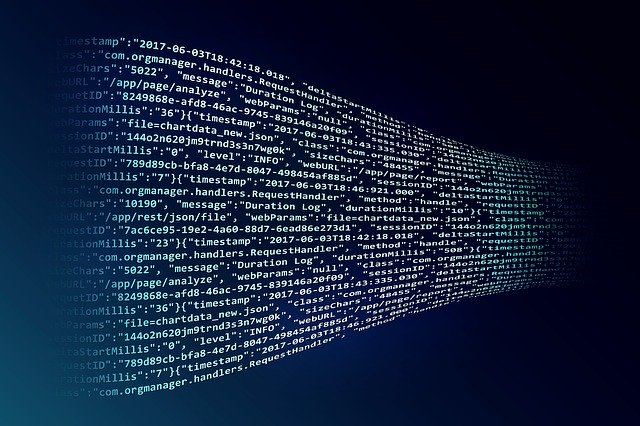
- Citation Author(s):
-
Harshita SKiran GangoorManoj S
- Submitted by:
- Manoj S
- Last updated:
Abstract
The proposed system utilizes eight ultrasonic sensors, with one sensor detecting maximum distance and another detecting minimum distance along each path at a 4-way intersection. Picture a bustling intersection where the proposed system, armed with eight ultrasonic sensors strategically positioned along each pathway, monitors traffic density in real-time. As vehicles approach, these sensors gauge both maximum and minimum distances, providing crucial data for dynamic signal timing adjustments. For instance, when heavy congestion looms, the system intelligently extends green signals for less congested lanes while shortening them for congested ones, effectively managing traffic flow. Moreover, with sound sensors tuned to detect ambulance frequencies, the system swiftly prioritizes emergency vehicles, promptly switching their designated lanes to green to expedite their passage. This seamless integration not only optimizes traffic flow but also enhances emergency response times, exemplifying a holistic approach to intersection management that prioritizes both efficiency and safety.
In our project leveraging ultrasonic sensors for traffic management, the data collection process begins with strategically placing these sensors along roads or at key points to monitor traffic flow. Ultrasonic sensors emit high-frequency sound waves, and by measuring the time it takes for these waves to bounce back after hitting an object, they provide precise distance measurements. These measurements offer insights into the proximity of vehicles or obstacles to the sensors, forming the foundational data for our traffic analysis.
The data collected from ultrasonic sensors primarily comprises distance measurements, which essentially reflect the distance of vehicles or objects from the sensors themselves. This information is invaluable for understanding various aspects of traffic dynamics, including vehicle density, speed, and potential congestion points. By continuously gathering and processing this data, we gain real-time insights into the state of traffic in the monitored area. Before utilizing this data for training deep learning models, it undergoes a preprocessing stage.