Analysis
Dynamic assessment and prediction of equipment status in 220kV substations by big data and AI technology
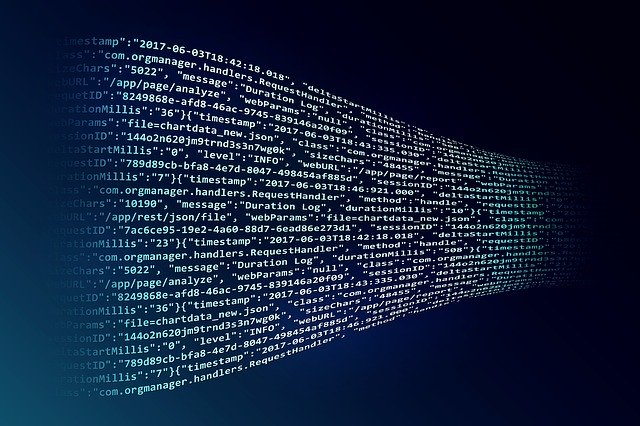
- Citation Author(s):
- Submitted by:
- hongshun Liu
- Last updated:
- Sun, 01/26/2020 - 11:09
- DOI:
- 10.21227/hxkm-2q16
- License:
- Creative Commons Attribution
Abstract
The current paper aims to present new hybrid model of fault classification based on implementing the principle of data reduction for Dissolved Gas Analysis data (DGA) of power transformers by using the combination (subsets) of set (C-set) method and the unsupervised FCM clustering algorithm. We used Multiclass Support Vector Machine (MCSVM) for performing the final classification. Bearing in mind the compactness of DGA data, C-set method is employed for dividing the data samples according to their fault types into multiple groups without repetition. FCM algorithm is adopted for samples pre-selection process by obtaining the cluster centers of each C-set class in order to reduce the dimensionally size of the DGA data set and the uncertainty of the transformer condition monitoring. Then, 1-vs-1 MCSVM method with linear kernel, has been used to carry out the fault diagnosis and perform the final classification. The proposed model diagnosis obtained accuracy, is 88.9%. Our proposed model has been compared with other soft computing techniques and traditional models. The proposed experimental model results revealed high performance in classifying transformer faults, improving the fault identification accuracy and shortening the computing time. Our new model is straightforward and accurate, suggesting good prospect of use in engineering practice.