Dataset Entries from this Author
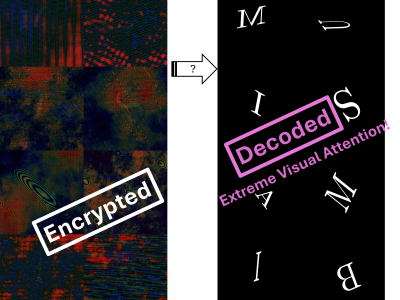
The dataset provides a collection of image-based classification and regression problems for artificial intelligence, under extreme visual attention constraints. Spatial shapes with simple geometries and called geometrons are embedded in an intense visual texture stream, with the aim of investigating the limits of artificial visual attention to capture known or unknown, but ghost or buried shapes.
- Categories:

The "Noisy Imperfect Partial Symbolic Sketches" (NIPSS) dataset provides symbolic sketches generated from the texture extraction method described in [1] when the inputs are associated with, either Imagenet animals [2] that have been preprocessed by [3], or the CelebAMask-HQ person faces described in [4].
Any sketch is an image containing a multichannel (artificial color) binary sequence of information, where artificial colors have consisted in concatenating the results of different regularizers from [1].
- Categories:
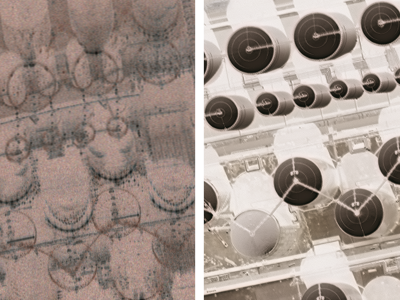
Remote imaging systems raise unprecedented challenges in artificial intelligence. The dataset provided (extracted from the SpaceNet 6 challenge) shows SAR images having distorted intensities (compared to the expected results, the latter being visible in the RGB and NIR images which are also provided) due to the geophysics of the SAR acquisition system and the geometries of ground objects. Can we teach an Artificial Intelligence to find the right re-projections for automatically correcting such distorted and compressed intensities ?
- Categories:

The dataset provides textures generated from elliptical cosine and sinc fractional Brownian field models.
- Categories: